Data-Powered AI in University Enrollment
Opportunities and Challenges
8/12/20232 min read
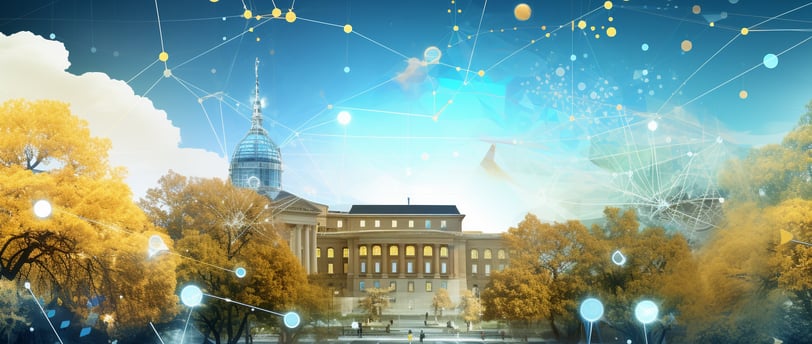
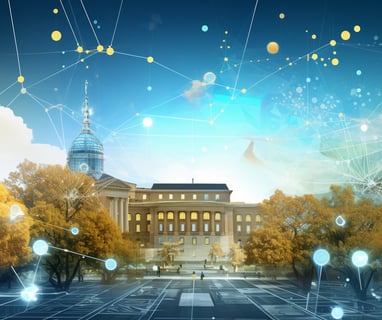
The integration of Artificial Intelligence (AI) into higher education has ushered in a transformative era for university operations, particularly in the realm of student enrollment. This article delves into the burgeoning role of data-powered AI tools in enhancing enrollment processes and streamlining university operations. While the potential of these tools is undeniable, the challenges associated with scaling and training warrant academic scrutiny.
Introduction
The digital age has seen the proliferation of AI across various sectors, with higher education emerging as a notable beneficiary. Universities, in their quest for efficiency and precision, have increasingly turned to AI tools, especially those powered by vast data sets. One of the most significant impacts of this integration has been observed in the domain of student enrollment.
The Role of Data-Powered AI in Enrollment
Predictive Analytics: AI tools, equipped with historical data, can predict enrollment trends, allowing universities to make informed decisions about course offerings, faculty allocation, and infrastructure investments.
Personalized Outreach: By analyzing prospective students' digital footprints, AI can tailor communication strategies, ensuring that outreach efforts resonate with individual preferences and interests.
Streamlined Operations: Manual tasks, such as sorting through applications, verifying documents, and scheduling interviews, can be automated, leading to faster and more accurate enrollment processes.
Challenges in Scaling and Training
While the advantages of AI in enrollment are evident, scaling these tools to cater to larger student populations presents challenges:
Data Privacy Concerns: As universities collect and analyze more data, concerns about student privacy and data security become paramount. Ensuring GDPR compliance and other data protection regulations is crucial.
Training and Development: Implementing AI tools requires a skilled workforce. Universities must invest in training staff to use these tools effectively and ethically.
Bias and Fairness: AI models, if not trained on diverse data, can perpetuate biases. Ensuring that enrollment algorithms are equitable and don't inadvertently disadvantage any group is essential.
Infrastructure Limitations: Scaling AI tools necessitates robust IT infrastructure. Many universities, especially those in resource-limited settings, may find this challenging.
Conclusion
The marriage of data-powered AI tools and university enrollment processes promises a future of efficiency, precision, and personalization. However, as with any technological integration, it comes with its set of challenges. As universities continue to tread this path, a balanced approach that harnesses the potential of AI while addressing its challenges head-on will be pivotal. The academic community, with its penchant for critical evaluation and innovation, is well poised to lead this charge, ensuring that the AI revolution in higher education is both impactful and inclusive.
Higher Education Dive
1, Olde English Ct, Romeoville, Chicago, IL
312-940-7076
info@higheddive.com